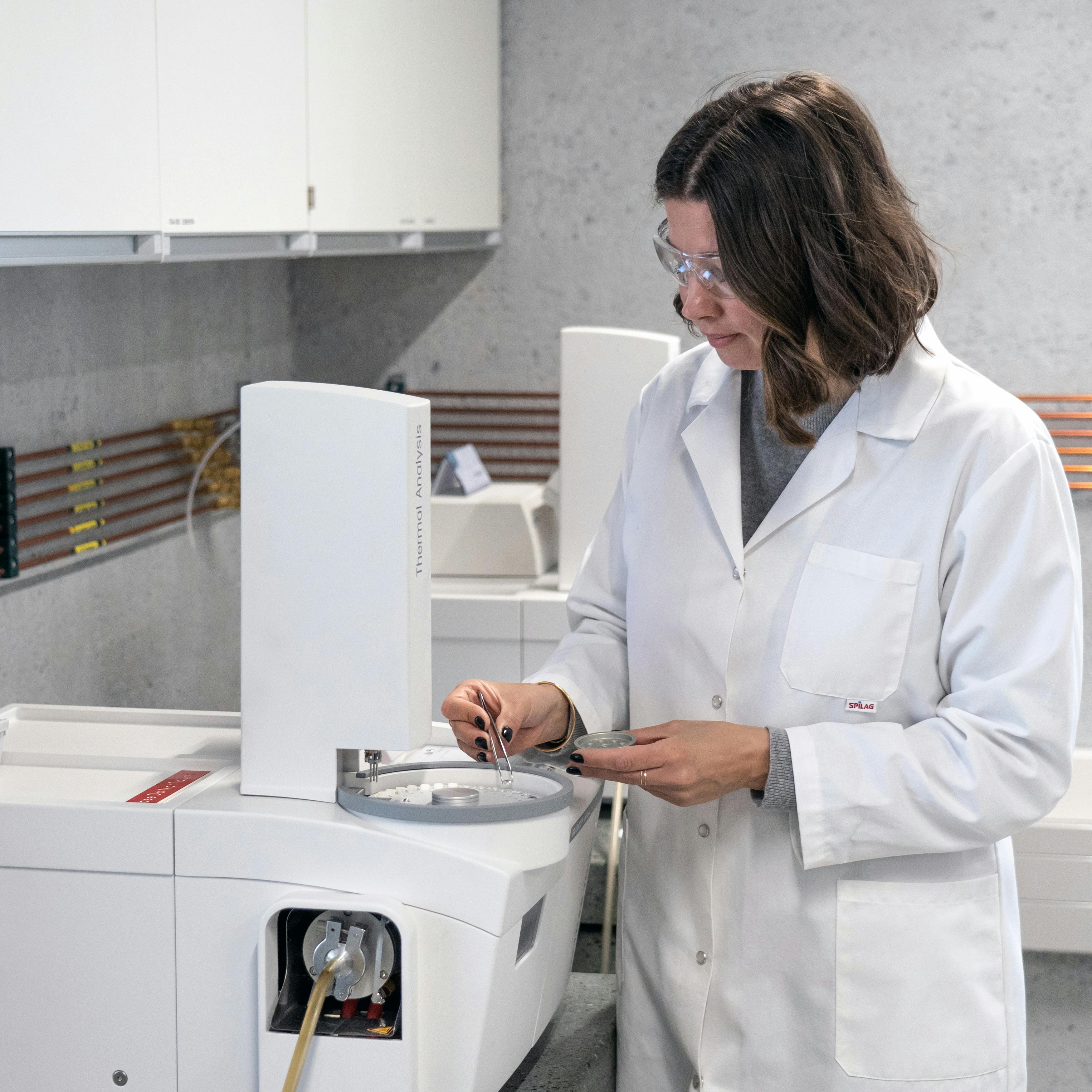
Using AI to advance DSC
Mettler-Toledo sent out a tender via the Data Innovation Alliance network to explore this idea’s potential, and several institutes and universities expressed interest. Mettler-Toledo created a consortium with leading research partners ZHAW and CSEM. ZHAW worked on the statistical methods and towards ensuring robustness through robust statistical significance analyses. CSEM contributed by drawing on its extensive knowledge and practical experience in deep learning within industrial applications.
Mettler-Toledo contributed its full domain expertise to the project and integrated the AI solution into their commercialized STARe software. Additionally, Mettler-Toledo processed a huge volume of expert data for the project based on numerous measurements that had previously been measured for publications and reference libraries.
ZHAW developed statistical methods for cleaning the data, analyzing expert data, identifying incorrect labels, and the robust automatic setting of measuring tangents. CSEM was tasked with developing the neural network and robust training algorithms including data augmentation and generation, as well as with validating and checking the results for plausibility.
The result of the project is the new software option AIWizard, which has already been integrated into the well-established STARe software. It facilitates the fully automated evaluation of thermoanalytical measurement curves of unknown samples.
Artificial intelligence: The path to success
Particularly challenging is determining the effect limits (where an effect begins and where it ends). The transitions are fluid in many cases, but exact positioning is essential to obtaining information about the type of effect (e.g., melting, crystallization, glass transition). This is where AI comes into play. The aim was to automate the complex and often error-prone part of the effect determination and thereby combining the knowledge and experience of many experts from different domains.
Experienced employees were initially highly skeptical. They claimed that "automated evaluation is simply not possible". However, in the end, the solution proved to be convincing, and it was concluded that the results far exceeded their expectations.
Neural networks are data hungry, which is why large amounts of expert data were needed. To further enlarge the data set comprising hundreds of measurements, thousands of items of artificial data were generated – in a process known as data augmentation and data generation. However, it is important to consider the ongoing physical processes. Data generation refers to the modeling of processes or the recombination of real data to generate new data sets.
"We have learned a great deal about how new technologies are benefitting customers today. Without the specialist knowledge of the project partners, we would not have been able to implement the idea," concludes Urs Jörimann, Mettler-Toledo project manager.
This project was funded by the Swiss Innovation Agency, Innosuisse (project number: 35056.1 IP-ENG).